Project leader: Prof. Dr. Andrea Bräutigam (University of Bielefeld, Computational Biology)
Email:Staff:
- Dr. Bart Verwaaijen
WP II.9 (Predictive power of regulatory network analyses) aims to exploit the predictive power of regulatory networks. The AVATARS project generates, analyzes and visualizes large datasets describing seed generation, seed filling and germination in the crop species rape seed (Brassica napus L.) in a 3-D AR and VR environment. Network generation and analyses provide a backdrop onto which the large datasets are mapped for detailed analyses and are visualized for intuitive, productive access.
The gene regulatory network (GRN) approach relies on machine-learned transcription factor – target gene associations. This approach requires large expression datasets generated from publicly available data and data internal to the consortium, as well as a defined set of regulators chosen based on their similarity with A. thalianaregulators. Using the regulators, a random forest decision tree regression approach defines the contribution of each regulator to the expression of a target gene.
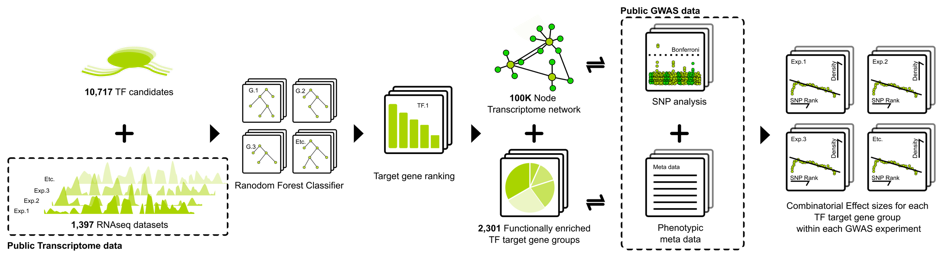
The method produces extremely noisy data with estimates of false positives of up to 80%. Careful data post-processing separates the “wheat from the chaff”. The functions associated with the transcription factors are similar to those assigned to homologous Arabidopsis transcription factors and thus validate the method. With additional data from within the project, networks are expected to be further refined, especially in regard to seed development and metabolism. The GRNs provide transcription factor candidates controlling the processes related to seeds. In addition to this primary role of the GRNs, they are also explored to (i) predict gene expression and to (ii) assist in GWAS data evaluation. The latter will also help to further filter false positive associations if a GWAS dataset corresponding to a particular condition of interest is available. The incentive thought being, that most evolution/adaptation occurs in cis and hence GRNs with their ability to define groups of genes dependent on particular transcription factors are hypothesized to improve the interpretation of GWAS results. Arabidopsis networks are used as proof-of-concept networks to test the usefulness of GRNs for a later transfer of the method to B. napus. The GRNs and their outputs are expected to be integrated into the 3D visualizations that will be generated by Breakpoint One GmbH as part of WP II.14 .